My top 5 takeaways from Build a Career in Data Science (Growing into your data science role) β PART 4
Posted on: May 9, 2024
Post Category: Book Notes
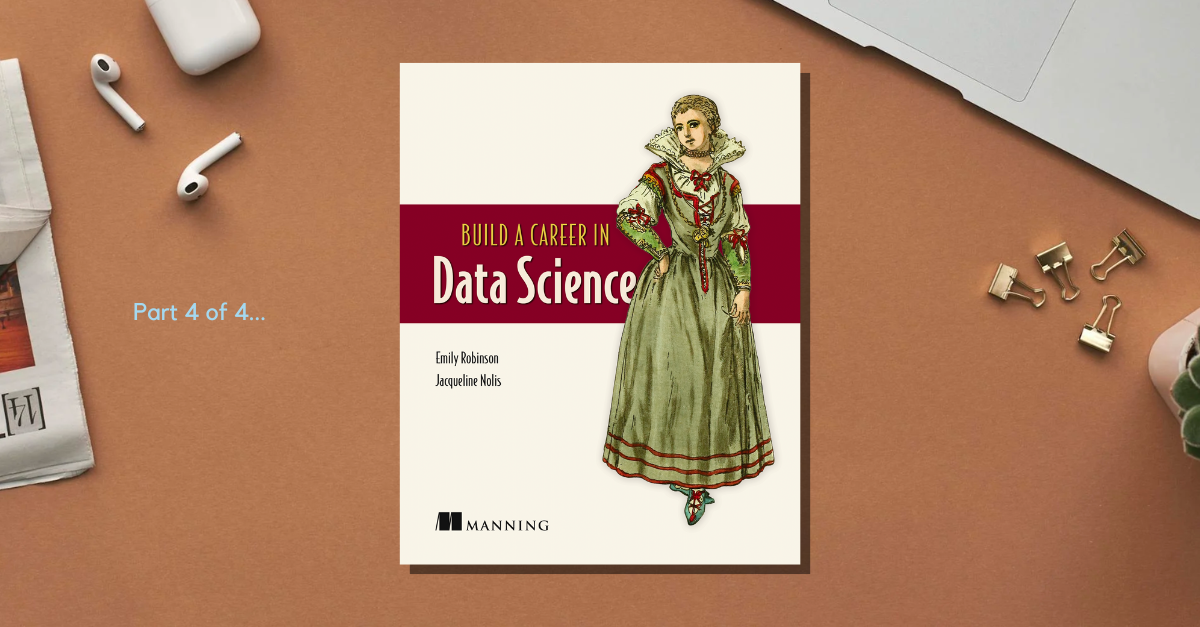
‘Build a Career in Data Science’ helped me understand and apply for data analytics jobs.
And if you’re more interested in that aspect, I’ve attached links to my notes on those two parts here:
Getting started with data science (part 1)
Finding your data science job (part 2)
The latest part I wrote about also happened to be part 3 (Settling in data science) and you can read it here.
For this particular blog post, I’ll be focussing on the fourth and final part of the book: Growing into your data science role.
What I found most relevant when reading the book was chapter 14: Joining the data science community (since I was, and still am, a junior data analyst).
But there are three other chapters in this part – about leaving your job, moving up the ladder, etc. – and I’ll offer coverage of all of them.
So here are the 5 key takeaways I took from this part of the guide:
1. Reflect on failed data projects – and know how to mitigate common issues
A project fails when it doesnβt meet its objective – an analysis doesn’t help the stakeholder answer a business question, a model isn’t deployed or doesn’t work as expected, etc.
But understanding how data science projects fail will help you avoid them in future.
Some ways they fail include:
- The data isn’t what you wanted: it doesn’t exist, it’s not stored in a useful format, etc. In this scenario, try to get samples of data, and if that is not possible, work on a project timeline designed around the possibility that the data will be poor – which in that case, find substitutes, actually begin collecting useful data, etc.
- The data doesn’t have a signal: in actuality, it’s easier to find no signal than a signal. And not having a signal could mean the end of a project. But you get out of it, you can reframe the problem to see if another signals exists, change the data source. One thing to keep in mind is to start with simple modelling methods to detect signal – complex ones can come later if these is one.
- The customer did not end up wanting it: Avoid this mistake as much as possible by taking the time to talk and work with your customers – so you understand their needs, their desires and their problems. When you do, you’re more likely to create something that they will use.
But in the case where a project actually fails, here’s what you should do:
- Document lessons learnt: ask yourself why it failed, what could have been done to prevent the failure, and what did you learn about the data and the problem. This helps you find/diagnose the problem, ways to avoid to similar situations in the future (e.g., have an exploratory phase if data wasn’t good enough), and what you can salvage from the project for other alternative project ideas. If pivoting is not an option, consider ending the project and being fully transparent/communicative with your stakeholder.
- Consider pivoting the project: this means repurposing the project or current progress into something that might succeed
- End the project and communicate with your stakeholders: If pivoting is not an option, consider ending the project and being fully transparent/communicative with your stakeholder. This frees time and space for more promising work.
2. How to manage risk and maintain credibility
One big consideration with risk is how many projects you are working on simultaneously. If you are working on a single risky project, it can be hard to handle careerwise if it fails.
Having multiple projects can be beneficial here; if you’re stuck on one, you can make progress on another.
Another way to manage risk is by baking early stopping points into a project – i.e., design project timelines with the expectation that it fails past a certain point. For example, a project can be stopped after you spend a number of weeks searching for the data – if it’s unclear that the data exists.
Any sufficiently interesting project is going to have plenty of uncertainty and unknowns – new datasets, new methods, new stakeholders, etc.
Also remember that being a data scientist is like being a treasure hunter – a junior data scientist is like a novice treasure hunter looking for standard goods, while a senior data scientist is like a experienced treasure hunter looking for greater treasures. Sometimes the analysis works, sometimes it doesn’t.
3. How to join the data science community
You can advance your career in other ways than doing well at your job. Presenting talks, contributing to open source, attending conferences, growing your portfolio are other ways – and they are all about engaging with the data science community.
Here’s more detail about the four activities:
- Growing your portfolio: writing more blog posts and creating more projects (in your own time)
- Attending conferences: an opportunity to network with other professionals in the space, put your company’s name out there and learn about techniques that could help you solve a problem at work
- Giving talks: this is accessible after you make a proposal for a conference/meetup (and you don’t need to be an expert in the field)
- Contributing to open source: contributing to other people’s work online, like writing packages documentation, and making packages public
Four key benefits of doing these activities:
- Gaining skills – learning skills that you wouldn’t have learnt while working your day job.
- Growing your network – gaining access to people with similar/greater expertise.
- Getting opportunities – i.e., you will more likely be asked to help on projects, give talks, or speak on a podcast, and you might find your next job from someone you meet
- Giving back – which can make your job feel more fulfilling
4. How to leave your job gracefully
Three parts to consider:
- Deciding to leave
- Starting the job search
- Giving notice
And here are things to consider for each part:
- Deciding to leave
- Ensure that you are always learning – see opportunities to improve you nontechnical skills and learn from adjacent teams, before you consider a new role
- Tell your manager what you would like to change – an unsolvable issue at work might have a solution
- Starting the job search
- List what you enjoyed in your current role – and find a company that values the same things as you or has the structure you’re looking for
- Think about roles about that will set you up for success in the long term
- Protect your market role – roles with the data scientist title generally get paid more than data analysts
- Be prepared to be asked “why are you leaving your job?” – and have a good answer prepared. Generally this would look like that you’re interested in some specific project work or that you’re looking for challenges.
- Be prepared to be asked questions you don’t know the answer to – admit it, or talk about how other experiences might support you with the tool/skill at hand. For example, a role might require Python, but your years of experience with R can be transferrable.
- Try to continue to do good work – you may need a recommendation from your manager one day, and that company is still paying you.
- It’s OK to call off your job search.
- Giving notice
- Give at least two weeks notice and discuss with your manager during your one-on-one or career discussion with them
- Assure that you will help with transitioning
- You may be given a counteroffer – which you could accept but you will be seen as a flight risk, and you might strain the relationship with your manager.
- Give people a way to contact you after you leave (email, LinkedIn, Twitter, etc.)
- Add tutorials, organise files (in shared folders), and add comments and explanations to analyses
- Check that you are saving documents relating to your offer and departure from the company.
5. The different options you have available when you move up the ladder
There are three options:
- Manager
- Technical lead (or principal data scientist)
- Independent consultant
Here’s what they all entail:
- Manager
- People who lead teams, set strategy, and give career mentoring.
- Responsible for determining the team’s work, who should be on the team, mentoring team members, resolving team issues and managing projects.
- A great role if you want to climb higher ranks, and if you like teaching and helping others, but you don’t have time to do actual data science – you have to worry about the performance of your team.
- Technical lead (or principal data scientist)
- People who are masters of their field, who companies rely on to solve difficult problems.
- Responsible for influencing data science strategy, mentoring junior data scientists and finding solutions to difficult problems – being strong enough to lead others and handle full projects without outside guidance.
- You won’t have someone to turn to when you’re stuck, but you get the most interesting (though sometimes annoying) problems to solve.
- Independent consultant
- People who have enough skills and a large-enough network to be able to freelance for a living.
- You have to do many different things – like marketing your business, doing sales, executing the project, delivering the results, and managing the business.
- Independent consultants get to be their own boss and get the opportunity to choose data science projects, the approach and how to present the results. But it’s wildly stressful, can make you broke, you won’t have anyone to turn to, and you won’t be doing much data science work (more admin and finding clients).
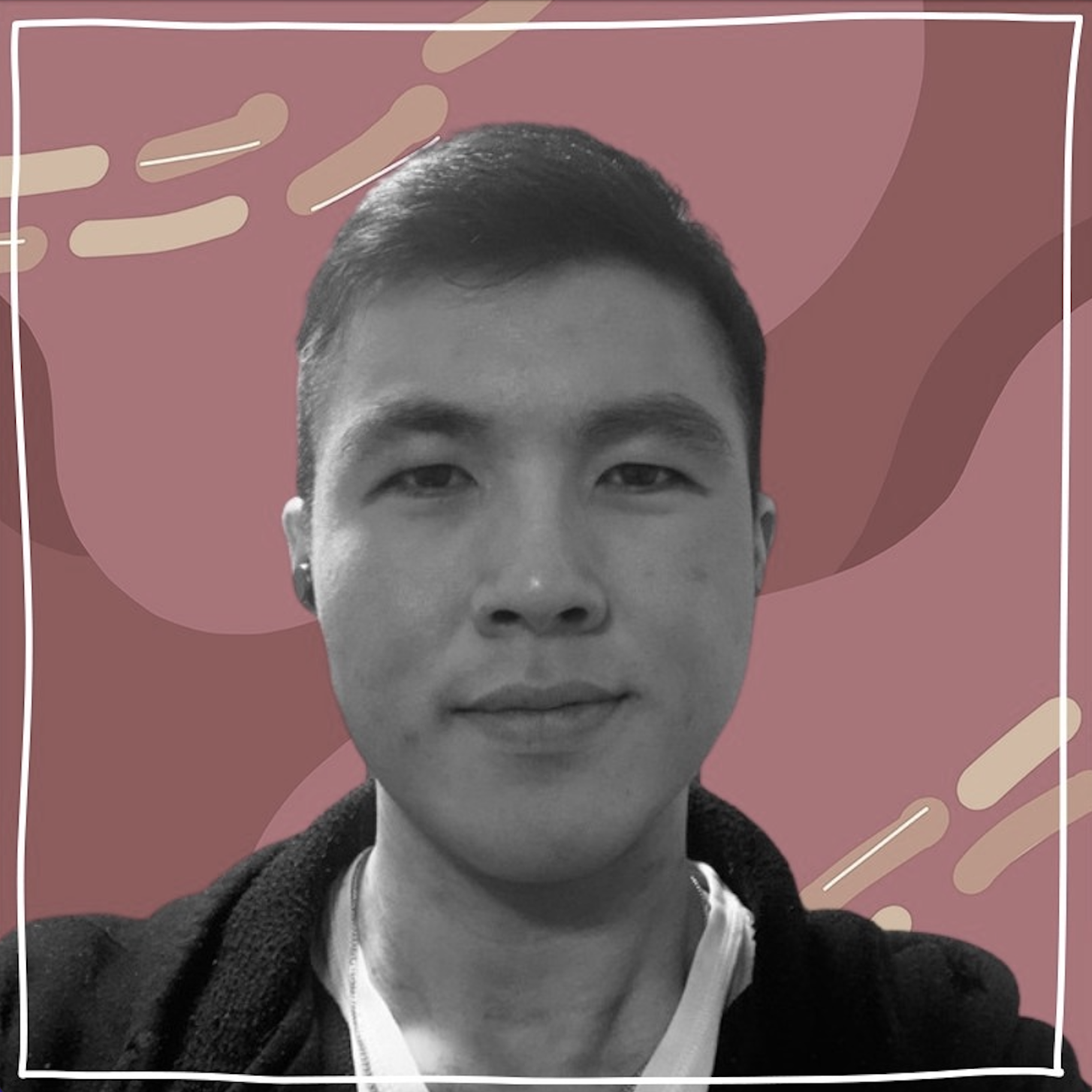
About the author
Jason Khu is the creator of Data & Development Deep Dives and currently a Data Analyst at Quantium.