My top 5 takeaways from Build a Career in Data Science (Finding your data science job) – PART 2
Posted on: February 25, 2024
Post Category: Book Notes
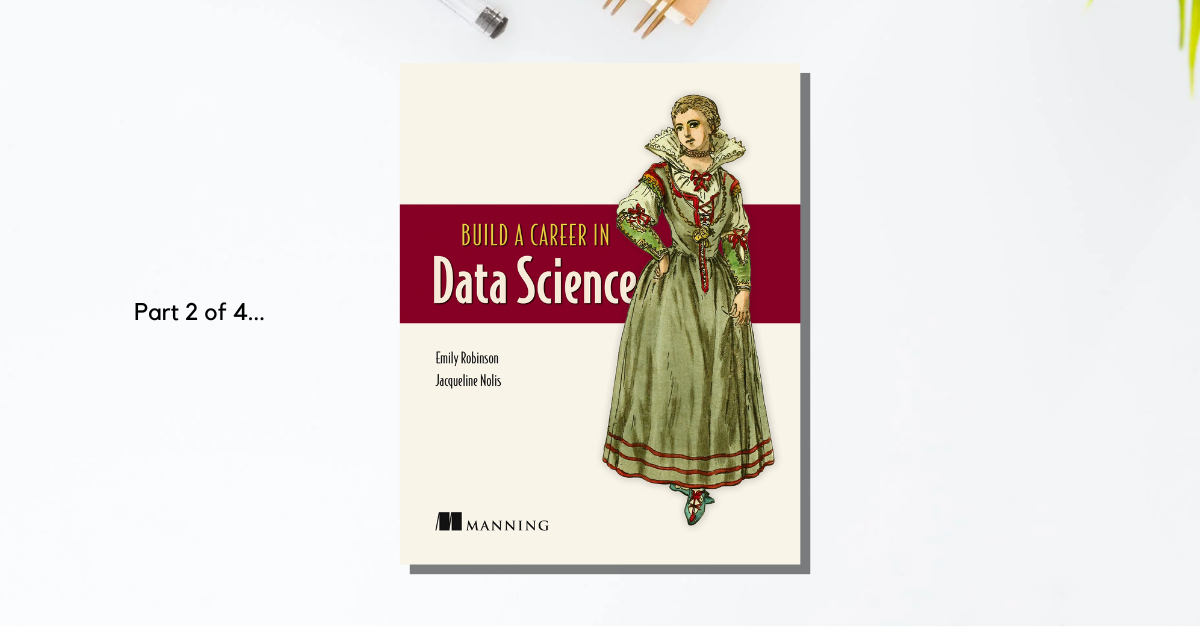
‘Build a Career in Data Science’ is one of the most consequential books I’ve read for my growth in the data analytics space.
And this particular part of the book was the most helpful.
For this blog post, I’ll be focussing on the second part of the book: Finding your data science job.
A lot of my resume-writing practices and cover letter-writing practices come from this specific part of the book.
There are samples/templates I keep referring to even today, when writing my own stuff – and even when reviewing other people’s analyst resumes.
It was like a guiding light when I didn’t have one.
But anyway, enough about my personal experience with the content…
Here are 5 key takeaways I took away from this part, and I hope you find them useful too:
(Note there is a chapter in this part of the book dedicated to application processes – case studies, technical interviews, etc. With the exception of applying of the role, I have left that out (for now). The following takeaways were what I found to be most relevant for myself and analysts earlier in their journey.)
1. Increase your surface area of success – by building your network.
The two most common ways that people, who are already employed as data scientists, look for and get jobs is through recruiters and friends, family members, and colleagues. So building your network is important here.
A good way to build that network is by going to meetups.
Meetups are in-person meetings held on weekday evenings. There’s usually a speaker, panel, or series of speakers presenting on a topic that’s relevant to the event.
Many data science meetups have time at the beginning/end for people to announce whether they’re hiring. Go up and talk to those people.
Even if their current openings aren’t a good fit, they may be able to give you good advice or suggest other places to look.
Another way to start building your network is by using social media platforms, like Twitter and LinkedIn.
Go public with your job search and start making a name for yourself:
- Share your work – like a project or blog post.
- Share other people’s work – share their work, mention the benefit you received from their work, and tag the creator.
- Ask for help – on any issues you’re having trouble solving (even after a Google search).
- Share tips – because even experienced analysts/coders have something new to learn.
But generally, building your network will prepare you for the next time you look for a job – be it at conferences, meetups, academic institutions, barbecues, etc.
2. Know the resume essentials
For your contact information:
- Include your first/last (common) name, phone number and email at minimum.
- Try share links to your social media profile or online pieces of work – LinkedIn, GitHub, personal websites/blogs.
- Don’t use email addresses that are offensive or might expire.
For your experience:
- Include previous jobs, internships, research jobs and bootcamps.
- Mention the position you’ve held, company name, the month and year of your start and end, your job title and at least one bullet point describing your work/impact.
- Spend more time on relevant experiences (by writing closer to 3 dot points).
- This section should be the largest, potentially taking up half the space.
For your education:
- List your school(s), dates and area of study.
- If you’re a recent graduate, you can list relevant coursework like statistics, mathematics, or computer science.
- If you’re a recent graduate and your grade is high, you can list it.
For your skills:
- Include at most 8 skills that are either programming/database skills or data science methods.
- Avoid star ratings and listing soft skills.
For your data science projects (optional):
- Include a title, descriptions of what you did and how you did it, and the results.
- Include a link to a blog post or GitHub repo with an informative README file.
For your publications (optional):
- You can briefly include published papers that are in quantitative fields but not directly related to data science (but only briefly).
And finally, have clean formatting and proofread your resume.
3. Know the cover letter essentials
If you need to write one, here’s a standard structure you can follow:
- Greeting. Do your best to find out who the hiring manager or recruiter is, and address the letter to them. If no luck, address the letter to “[Department Name] hiring manager”.
- Introductory paragraph. Introduce yourself, name the position you’re interested in, and briefly explain why you’re excited about the company and role – ideally referring to any content and pieces of work shared by the company or their individual data scientists.
- One to two paragraphs of examples of data science work. Connect your previous accomplishments with this role. Add details to the things you discussed in your résumé.
- Closing paragraph. Thank the recruiter for their time and consideration. Sum up your qualifications by explaining why you’d be a good fit for the position.
- Signoff. Close with “Sincerely,” “Best,” or “Thank you for your consideration”.
4. Know how to do more than the basics – tailor
Use keywords from the job description, and keep the language as simple as possible.
The first person who screens your résumé isn’t likely to be the manager for the position; it may not even be a human! At larger companies, applicant tracking systems automatically screen résumés for keywords, surfacing those that contain the keywords.
You want someone to be able to look back and forth between your resume and job description easily, finding exact matches for the requirements in your experience; if you use too much jargon, and the reviewer is not from the same technical background, it’s harder to figure out if you’re a match.
If you’re applying for big tech firms or well-known, fast-growing startups, you usually have to (express that you) meet 90-100% of the requirements. Because oftentimes, these companies are looking for reasons to reject people.
5. Know how to do more than the basics – ask for a referral
Companies like people who are referred because they come pre-vetted: someone who already works at the company and (presumably) is doing well thinks that this person would be a good fit.
Even if someone doesn’t formally refer you, being able to write in your cover letter “I discussed this position with [Star Employee X]” and have that person tell the hiring manager to look out for your résumé is a huge benefit.
Use LinkedIn to see whether you know anyone at the company and reach out with a polite message – you’re more likely to get a response.
Second-degree contacts are also good if you have something in common. You can get your mutual connections to introduce you.
In the next part, you’ll learn how to handle the early months of your data science job.
Stay tuned (or check out my more recent posts, if it’s been shared already)!
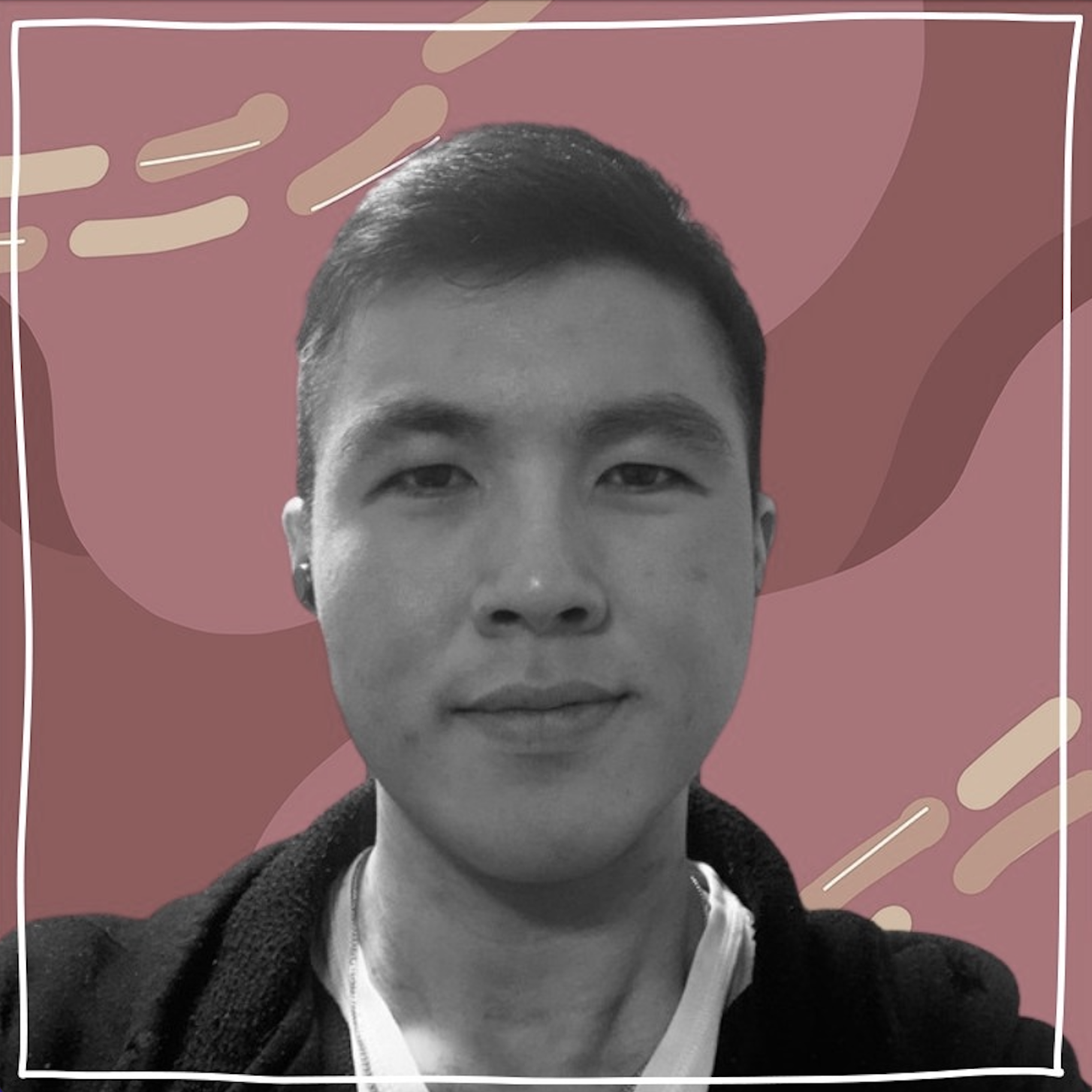
About the author
Jason Khu is the creator of Data & Development Deep Dives and currently a Data Analyst at Quantium.
Notice:
Let me know if you want a resume review from me.
I have written a Data Analyst resume checklist, which will be free for you to read when my Data Analyst Guide gets released on the 3rd of June, 2024.
But if you want specific feedback from me, delivered in a short 30-minute to 1-hour call...
Please let me know through LinkedIn, and I'll see what I can arrange!
Pricing TBD.
- Jason